It's all well and good to talk big about finding traces of life on Mars, but we also need to know where to look. Getting to Mars is hard – we want to make sure to make the best use of the opportunities available so as not to waste the trip.
But there's a lot of ground to cover. Mars has almost the same surface area of dry land as Earth, with one key difference. Throw a rock on Earth, and it'll probably land somewhere with life. However, the history of life on Mars is a great big question mark.
Artificial intelligence and machine learning could make the search for life on Mars much less arduous. An international team of researchers led by astrobiologist Kimberley Warren-Rhodes of the SETI Institute has shown that these tools can identify hidden patterns in geographical data that could indicate the presence of signs of life.
"Our framework allows us to combine the power of statistical ecology with machine learning to discover and predict the patterns and rules by which nature survives and distributes itself in the harshest landscapes on Earth," Warren-Rhodes explains.
"We hope other astrobiology teams adapt our approach to mapping other habitable environments and biosignatures. With these models, we can design tailor-made roadmaps and algorithms to guide rovers to places with the highest probability of harboring past or present life – no matter how hidden or rare."
There's one place on Earth that bears a startling resemblance to the arid plains of Mars. That's the Atacama Desert in Chile, one of the driest places on the planet, not seeing rain for up to decades. Even in this inhospitable place, life can be found tucked away in pockets and under the ground.
Warren-Rhodes and her colleagues focused on a region at the boundary between the Atacama Desert and the plateau of Altiplano called Salar de Pajonales. This basin is an ancient riverbed and one of Earth's best Mars environment analogs. At 3,541 meters (11,617 feet), it's high in altitude and subsequently receives high UV exposure. It's also low in oxygen and extremely dry and salty… yet somehow, life can be found there, living in mineral formations.
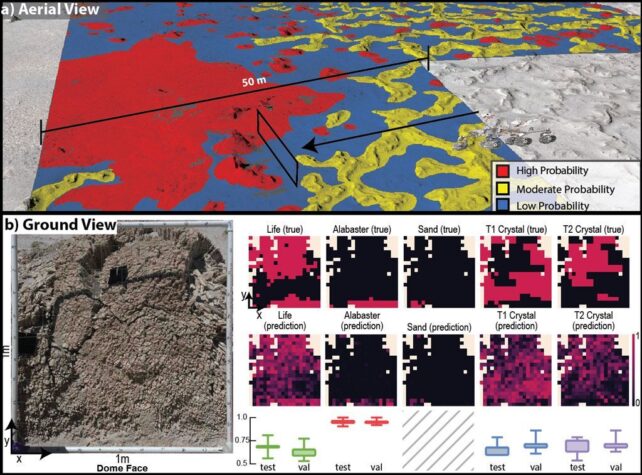
Over an area of 2.78 square kilometers (1.07 square miles), the researchers carefully took 7,765 images and 1,154 samples, looking for the telltale biosignatures that gave away the presence of photosynthetic microbes. These included carotenoid and chlorophyll pigments, which tint the rock pink or green.
They also used drones to take aerial images to simulate images obtained by the satellites that orbit Mars and added 3D topographic maps. All this information was then fed into convolutional neural networks (CNNs) to train the AI to recognize structures in the basin more likely to be teeming with life.
And interestingly, the CNNs were able to identify patterns in the distribution of microbial life in the basin, despite the area's near-uniform mineral composition.
Domes of the soft mineral gypsum were around 40 percent inhabited, and patterned ground striped with ribbons of gypsum was about 50 percent inhabited. Taking a closer look at what parts of these features were inhabited, the researchers found microhabitats. The microbes were powerfully drawn to sections of alabaster, a fine-grained, porous form of gypsum that retains water.
These alabaster microhabitats, the team found, were "almost universally inhabited" and represented the most reliable predictor of biosignatures, suggesting that water content is the primary driver for microhabitat distributions.
More pertinently for the search for life on Mars, the CNNs enabled the researchers to correctly identify biosignatures up to 87.5 percent of the time, compared to up to 10 percent for random searches. This reduced the amount of ground they needed to cover by a whopping 85 to 97 percent.
"For both the aerial images and ground-based centimeter-scale data, the model demonstrated high predictive capability for the presence of geological materials strongly likely to contain biosignatures," says computer scientist Freddie Kalaitzis of Oxford University in the UK.
"The results aligned well with ground-truth data, with the distribution of biosignatures being strongly associated with hydrological features."
The approach, therefore, seems to have multiple benefits. The work taught us something about life in extreme environments here on Earth and shows promise for identifying life on Mars. And could help identify other biosignatures here on Earth.
The team plans to try to train their CNNs on other biosignatures, such as stromatolites, which are fossilized microbial mats that can be billions of years old, and communities of halophiles, organisms that thrive in super-salty environments.
"Our framework allows us to combine the power of statistical ecology with machine learning to discover and predict the patterns and rules by which nature survives and distributes itself in the harshest landscapes on Earth," says Warren-Rhodes.
"We hope other astrobiology teams adapt our approach to mapping other habitable environments and biosignatures. With these models, we can design tailor-made roadmaps and algorithms to guide rovers to places with the highest probability of harboring past or present life – no matter how hidden or rare."
The research has been published in Nature Astronomy.