In a continued effort to catch neurodegenerative diseases early, researchers directed machine learning models to see if they could 'hear' Parkinson's disease in patients' voices.
"Our findings suggest that voice-based machine learning models can detect disease signatures even before overt motor signs appear," University of North Texas bioinformatician Aniruth Ananthanarayanan and colleagues explain in their research, which is yet to be published.
Parkinson's impacts almost 9 million people globally. It is characterized by difficulty controlling fine movements and tremors in a patient's extremities, but it also causes challenges in people's moods, thinking, and memory.
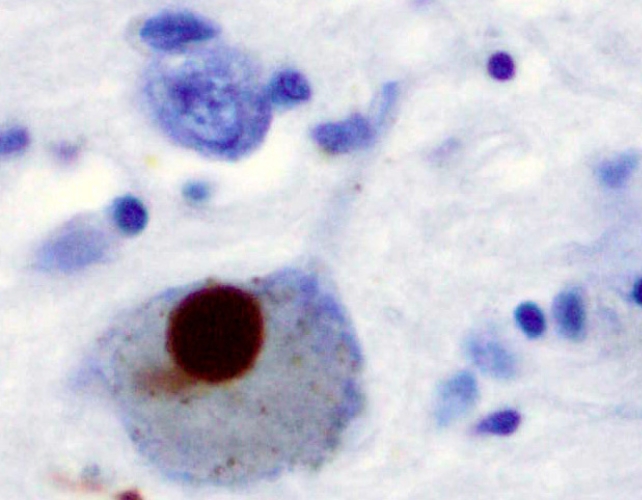
While the mechanisms behind the condition are more or less known, the triggers for the breakdown in function are yet to be fully understood. Everything from processed food to pesticides used on golf courses have been implicated, and there's also a genetic component.
There is currently no cure for Parkinson's disease, meaning the best patients and their loved ones can hope for are therapies that slow symptoms. The earlier such treatments begin, the more benefits they provide.
So, early detection can have a massive impact on a patient's quality of life.
Ananthanarayanan and his team used machine learning models to determine whether a selection of volunteers have Parkinson's disease from their voice alone.
They tested and trained their models 195 voice recordings from 31 people. Of these, 23 were diagnosed with Parkinson's. The pattern-seeking program accurately identified patients with the condition in 90 percent of its attempts.
The vocal features assessed by the models include the presence of a jitter, which is the result of irregular vocal cord vibrations; noise-to-harmonic ratios, a sign of the glottis not closing properly; and a measure of disordered voice signal patterns.
These traits have previously been linked to well-established symptoms of Parkinson's disease, including a hoarse voice, speaking difficulties due to weak vocal muscles, and a slowness or staggering in movement.
"Vocal symptoms like dysphonia [are] underutilized despite their diagnostic potential," the researchers explain.
They caution that further work is required to test their models' generalization, as the programs were trained using vocal data from only 31 individual people. This makes it unlikely their method could capture the full range of real-world voice differences across different ages, accents, and environmental conditions.
Data scientist Aiden Arnold, who was not involved in the study, told Clarissa Brincat at New Scientist this voice-based approach "shows real promise as an early screening too."
If the findings remain consistent across wider populations, such a tool would be an easily scalable and affordable option for early screening, as case numbers continue to increase.
This research is still awaiting peer review and has been uploaded to MedRxiv.