We don't yet have a cure for Alzheimer's disease, but detecting it earlier means preparations and perhaps even preventative measures can be put in place.
New artificial intelligence (AI) models could soon provide an early warning for individuals destined to develop the condition's symptoms years before they appear.
A team from the University of California, San Francisco (UCSF) and Stanford University applied machine learning methods to more than 5 million health records, training the AI to spot patterns that connect Alzheimer's to other conditions.
The resulting system isn't perfect, but when tested against records for people known to have developed Alzheimer's later, the AI was able to accurately predict its development 72 percent of the time – up to seven years prior, in some cases.
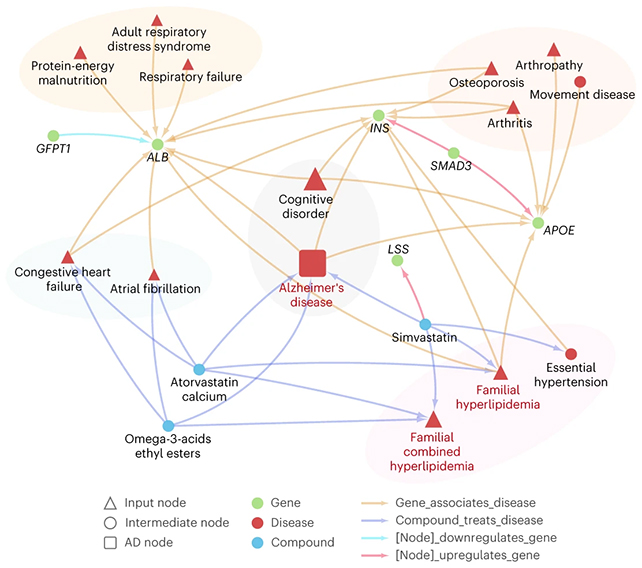
The AI system's predictive power stems from its ability to combine analyses of several different risk types to calculate the likelihood of Alzheimer's developing. The findings could tell us more about the disease's causes, as well as who might be vulnerable to it.
"This is a first step towards using AI on routine clinical data, not only to identify risk as early as possible, but also to understand the biology behind it," says bioengineer Alice Tang, from UCSF.
The model detected a number of conditions that could be used to calculate Alzheimer's risk, including high blood pressure, high cholesterol, vitamin D deficiency, and depression. Erectile dysfunction and an enlarged prostate were also significant factors in men, with osteoporosis (a skeletal disorder) significant for women.
That's not to say people with these health issues will develop dementia, but the AI analysis weighs each as predictors worth looking at. It's hoped that the same kind of machine learning approach might one day be able to identify risk factors for other hard-to-diagnose diseases.
"It is the combination of diseases that allows our model to predict Alzheimer's onset," says Tang. "Our finding that osteoporosis is one predictive factor for females highlights the biological interplay between bone health and dementia risk."
The researchers also investigated the biology behind some of the identified links. Osteoporosis, Alzheimer's in women, and a variant in the gene MS4A6A were found to be connected, providing new opportunities to study the disorder's development.
"This is a great example of how we can leverage patient data with machine learning to predict which patients are more likely to develop Alzheimer's, and also to understand the reasons why that is so," says Marina Sirota, a computational health scientist at UCSF.
The research has been published in Nature Aging.